Developing a Table Games Analytics Strategy
Data is all around us. In fact, the amount of data being produced is doubling every 3 years per a recent IBM report. This explosion in data created a need to analyze it and a growing field – analytics.
Analytics is the discovery, interpretation, and communication of meaningful patterns in data. Although the definition is precise, the word as it is used can mean different things to different people. This article attempts to outline a useful framework to think about analytics and how that can be applied to table games.
What Do We Mean by Analytics?
Analytics can be categorized into following four groups, each of which is more valuable and more complex than the previous category to produce:
Descriptive Analytics: Analytics of this type typically answer the question “What happened?” Eg. What happened to my win this month vs the same month last year?
Diagnostic Analytics: These types of analytics typically address the question “Why did it happen?” Eg. Why was my win lower by 5% vs last year?
Predictive Analytics: These types of analytics predict/forecast future outcomes eg. “What will happen?”. The prediction usually uses historical data patterns to estimate future outcomes and then the business may be tasked with identifying actions to influence that outcome favorably. Eg. Given that my win is lower this month by 5% vs last year, what will it be next month?
Prescriptive Analytics: These types of analytics are action-oriented and address “How can we make something happen?”. This is the most valuable kind of analysis that identifies actions to be taken in order to achieve certain outcomes. Eg. How can I grow my win by 5% YoY for the remaining 6 months of the year?
Although every analysis is trying to answer the question “How can performance be improved?”, reality is that the further we are from prescriptive analytics, the more assumptions we must make and hence accept a lower probability of achieving desired outcomes. Let’s look at applying the framework for table game analytics.
Applying Analytics Framework to Table Games
As an illustration of a typical scenario for table games analytics, let’s assume the performance of Q1 for the current year is being reviewed:
Descriptive analysis will answer the question, what happened to my win vs last year and the answer could be win was lower by 5% vs Q1 prior year. While that is useful to know, it neither improves understanding nor aids in how to change this for the remainder of the year. This is an example of descriptive analytics and is prevalent in most organizations in the form of reporting.
Diagnostic analytics would dig deeper and ask the question, why was my win lower by 5% vs last year? The answer to that question could be that we saw a weakness in Baccarat segment. While this aids understanding of the cause of the soft performance, it provides only a vague guideline to improve performance i.e. let’s focus on Baccarat. It is also important to note that this category of analytics does not distinguish between controllable (and hence actionable) vs uncontrollable factors when it comes to causes.
Then there is the approach of predictive analytics which attempt to forecast what might happen in the reasonable future. Here we take current trends and project what the next month or quarter might look like. Since historical trends are being extrapolated, the same weakness for Baccarat will extend into the coming months leading to a similar conclusion as with the diagnostic analytics i.e. focus on improving Baccarat performance. Once again, there is not much specific actionable insight to improve performance that can be immediately acted upon.
Contrast the previous three approaches with the prescriptive approach to analytics. The question would not be what happened or why it happened, rather it would focus on how can we close the 5% gap between current trend and last year’s trend. This could then identify that we have been pricing Baccarat lower during high demand periods, resulting in missed yielding opportunities. Or maybe a new operating procedure resulted in a lower game pace which resulted in a lower win. Notice that this type of analytics focuses on actions that can be taken to specifically improve performance. They also allow us to go back and see if those actions were performed and measure the effects and compare them vs our expectations.
Conclusion
All of us would like to have prescriptive analytics. However, as the graphic below illustrates, the increased value in prescriptive analytics comes at the cost of increased complexity. So in order to get the most out of it, there has to be an iterative analytical process set up to leverage the output.
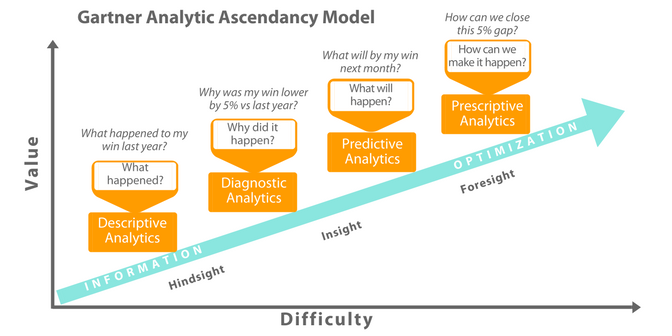
Building such a process allows us to cross the chasm from simplistic analytics that reveal superficial symptoms, to analytics that identify the deep-rooted causes with specific actions to fix them and improve performance – something we can all agree is the sole purpose of having analytics!

Request Access To The Full Webinar
Author(s)
Varun has over 15 years of experience in technology, finance, and casino analytics. Previously, he oversaw gaming optimization and established analytical processes to measure and improve profitability at Sands China in Macau and Caesars Entertainment in Las Vegas. Varun is a computer scientist by training and received his MBA from UCLA Anderson in Finance and Strategy.